This post is also available in Dutch.
What do the expenditures on science, space and technology in the United States have in common with the number of suicides by hanging, strangulation and suffocation? You guessed it: nothing! Except they correlate almost perfectly with one another (with a correlation score of 99.79%). This is a great example of a fake (or spurious) correlation: a strongly related pattern between two or more variables that are not causally linked; one does not cause the other.This is why correlation does not imply causation
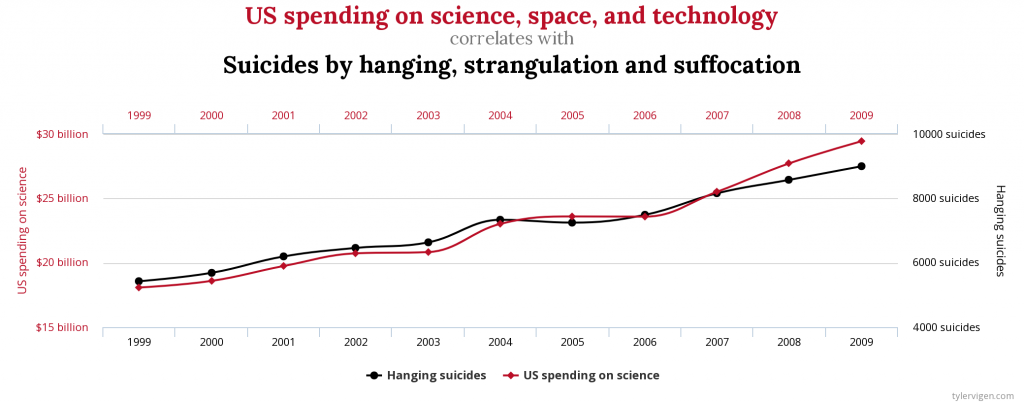
In this example, it’s quite clear that the relationship is meaningless. In science, however, there are examples of correlations that can be more easily interpreted as being causal (for example, ‘proof that people who drink coffee black are psychopaths’ in Dutch). Now, how can we confidently research causality in science?
Correlational versus experimental research
You might be tempted to think that with advanced statistical methods, we can easily infer causality, but that’s not the case. If we want to know whether psychopathic symptoms are actually caused by drinking black coffee, we have to carefully design an experiment to research this relationship.
There are roughly two types of experiments: correlational and experimental. We can only talk about causality in experimental experiments and that’s mostly down to two factors: manipulation (via intervention) and randomisation.
I will clarify these terms via the ‘black coffee causes psychopathy’ experiment.
To research this relationship in an experimental way, there has to be manipulation. This means that we, the scientists, measure the psychopathic symptoms of two groups of participants over a long period of time. One group will have to drink black coffee every day, whereas the other group drinks something else, for example, tea. If we only manipulate this one factor (what the participants are drinking), we can be more sure that differences in psychopathy are due to drinking habits. It is, however, extremely important that all other factors (e.g., participant traits such as age and gender) are similar across both groups.
For this experiment you could easily recruit people that you know only drink either black coffee or tea, but now you are at risk that other factors come into play: Maybe all people who drink black coffee are men and, by pure chance, it turns out that all men score higher on psychopathy.
To control such issues, an experiment needs to be randomised. We need to place participants in either of the groups at random and in advance. This way, we can exclude the influence of other variables (like gender).
Practical feasibility
In an ideal experiment, we are in full control of all factors and circumstances that could possibly influence the outcome of the experiment. To perform the above example perfectly, we would have to check everything that the participants eat and drink. You’ll understand that this is practically and ethically impossible. Some factors can’t even be controlled! Imagine that we suspect that lactose intolerance causes psychopathy. To perform an experiment to prove this, we would have to make one group of people lactose intolerant, which is—of course—impossible (and if we could, it would be highly unethical). Thus, our only choice is to accept that finding causality is not always possible and that correlational research is an important part of science.
In fact, correlations are used a lot in cognitive neuroscience (e.g. to research the relationship between brain activity and behaviour) and can (if applied correctly) lead to new insights. These new insights can then be used to further develop theories and make predictions. Overall, correlations aren’t all that bad.
Original language: Dutch
Author: Felix Klaassen
Buddy: Eva Klimar
Editor: Jill Naaijen
Translator: Wessel Hieselaar